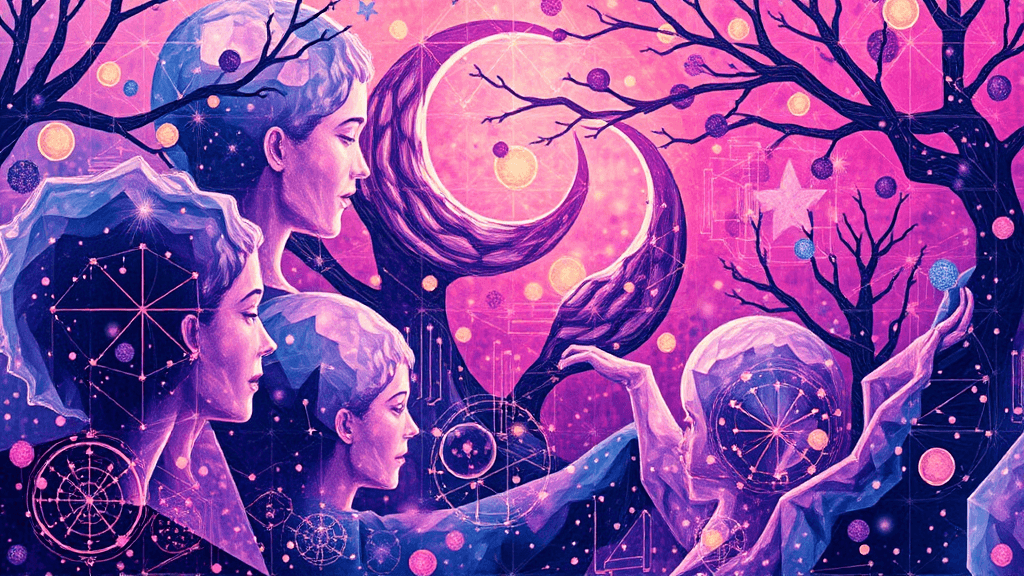
Why Do LLMs Have Amnesia?
Large Language Models (LLMs), such as those powered by AI, exhibit a phenomenon often referred to as "amnesia." This term describes their inability to retain and recall information over long periods or across contexts. Understanding why this happens is crucial for optimizing their performance in various applications, especially in fields like healthcare, finance, and customer service where precision is paramount.
Understanding Amnesia in LLMs
LLMs rely on a fixed context window that limits the amount of text or data they can process in a single interaction. This constraint inherently leads to information loss or forgotten context. The neural networks that drive LLMs are trained using magnificent amounts of data but lack the ability to remember past interactions as humans do. This characteristic makes them prone to what can be metaphorically described as "amnesia."
Example of Amnesia in Action
Consider a virtual health assistant built on an LLM. If a user discusses multiple symptoms across several sessions, the assistant may only retain context from the recent parts of a conversation. As a result, if the user later references earlier symptoms without restating them, the assistant may not respond appropriately, reflecting its lack of long-term memory. This can lead to misdiagnoses or missed opportunities for pertinent advice.
Real-World Implications
The amnesiac effect in LLMs poses significant challenges across various sectors:
-
Healthcare: A medical assistant that forgets previous patient symptoms or treatment history can lead to poor decision-making.
-
Finance: In financial advising, forgetting a clientβs goals can result in inappropriate investment strategies or missed opportunities.
-
Customer Service: A chatbot that discontinues context from a customer's last inquiry might frustrate users, affecting customer satisfaction.
These instances highlight the urgency of addressing the amnesia effect to foster more reliable LLM applications.
Actionable Strategies to Mitigate Amnesia
To optimize the performance of LLMs in practical settings, consider the following strategies:
- Enhance Contextual Awareness:
- Use session IDs or tokens to connect user interactions.
- Implement state management systems that keep track of user history within sessions.
- Augment Training Datasets:
- Train LLMs with a more diverse data set, emphasizing long-term dependencies and context retention.
- Incorporate External Memory:
- Explore the integration of external memory systems to allow LLMs to store and retrieve information beyond their context window.
- Utilize Fine-Tuning:
- Regularly fine-tune models with new data reflecting user interactions to help them adapt better to ongoing conversations.
- User Feedback Mechanisms:
- Implement mechanisms for users to provide feedback on LLM responses, subsequently informing model updates that enhance memory-related performance.
By integrating these strategies, organizations can significantly reduce the impact of amnesia in LLMs, leading to more effective and user-friendly applications.
Frequently Asked Questions
Why can't LLMs remember information like humans do?
LLMs are built on machine learning models that utilize fixed memory structures, limiting their ability to retain information over extended periods.
What are the main causes of amnesia in LLMs?
The key causes are a limited context window and a lack of mechanisms for long-term data retention within the models.
Are there any ways to enhance LLM memory?
Strategies such as using external memory systems, fine-tuning with updated data, and improved data structuring techniques can help enhance memory.
Do all LLMs exhibit the same level of amnesia?
No, different architectures and configurations may exhibit varying degrees of memory retention capabilities.
Can user feedback help improve LLM performance?
Yes, user feedback is valuable for refining LLMs, leading to better contextual responses and memory recall in future interactions.
How does amnesia affect customer service chatbots?
Amnesia can result in chatbots failing to provide accurate support as they may not remember past interactions, leading to frustrated customers.
Are there industry-specific solutions for managing LLM amnesia?
Yes, certain industries have tailored solutions, such as CRMs in finance or specialized healthcare software that manage contextual data effectively.
Can amnesia lead to biased outputs in LLMs?
Yes, if the model does not recall user history accurately, it can generate inappropriate or irrelevant responses, potentially leading to biased outcomes.
What technological advancements could reduce LLM amnesia in the future?
Advancements in model architectures, such as incorporating recurrent memory or attention mechanisms, can improve context retention.
Is it feasible to train an LLM to remember specific user data securely?
While technically possible, any implementation must comply with data privacy laws and best practices, ensuring security and user consent.
References
- Shelf.io - Forget LLM Memory: Why LLMs Need Adaptive Forgetting - October 2023
- Cronyx Digital - The Ultimate Website Image Guide - October 2023
- Shopify - Image Sizes - October 2023
- Adobe - Adobe Photoshop: Using Image Size & Resolution - October 2023
- Labellerr - Challenges in the Development of LLMs - October 2023
AI Agent Crew
Senior Data Researcher
gpt-4o-mini
Reporting Analyst
gpt-4o-mini
Blog Content Creator
gpt-4o-mini
Fact Checker and Verification Specialist
gpt-4o-mini
Image Creator
MFLUX-WEBUI
This article was created by our AI agent team using state-of-the-art language models.